Artificial Intelligence (AI) in Oncology
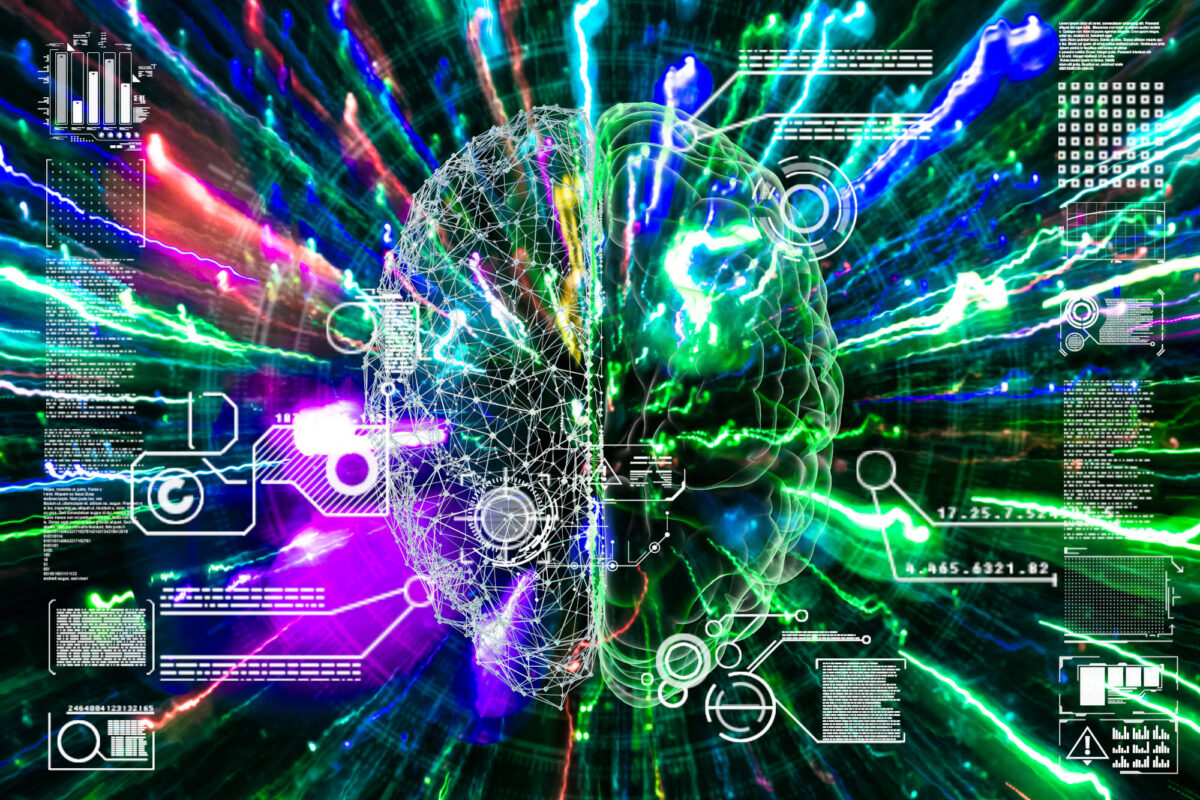
In the past decade, artificial intelligence (AI) and other smart technologies have permeated almost every area of society. At the same time, the concept of deep learning (DL) has revolutionized AI to make it more effective in its applications. Now, when given extensive samples of data, computers are able to make meaningful predictions and estimates which account for underlying patterns not yet recognized or coded for by humans. Therefore, fields that produce a lot of data—such as oncology— are the most attractive for DL applications. Currently, deep-learning AI is being used within oncology to improve diagnostic techniques, treatment methods, and disease management.
One area of oncology where AI has been especially successful is in image analysis. Given that imaging is a common practice in tumor detection and monitoring, a significant amount of oncological data is visual. While the field of visual data analysis is still relatively new, there have already been promising breakthroughs. For example, the Convolutional Neural Network (CNN), a newly-developed DL model, has been developed to analyze the orientation of each pixel within images, which leads to the appreciation of larger-scale objects.[1],[2] It was found that after being trained on 130,000 images, a CNN was able to detect malignant skin cancer with more sensitivity and specificity than a panel of 21 board-certified dermatologists.[3] Various CNNs have also been successful at segmenting tumor volumes, automating lung nodule detection/classification, and detecting breast cancer malignancy using radiographic imaging.[4],[5],[6],[7],[8]
AI has also shown a lot of clinical promise in the analysis of non-visual information. For example, DL algorithms have been able to predict genetic mutations from histopathological data— an assessment which may be useful in increasing detection of anomalies in oncogenes.[9] AI has also used information from the electronic health record to predict the development of diseases such as prostate, rectum, and liver cancer with 93 percent accuracy.[10] Since prognosis often depends on the stage in which cancer is detected, these predictive algorithms have the potential to improve the chances of survival and quality of life for future cancer patients. However, these algorithms have more than just preventative applications: they can also inform treatment plans. Artificial neural networks have been able to predict an individual’s likelihood to respond favorably to various treatments given genetic and chemical information.[11]
Given the host of applications for which AI has proven to be effective, including oncology, it is likely that DL algorithms will continue to be increasingly integrated as a part of cancer research as well as treatment.
[1] Krizhevsky A, Sutskever I, Hinton GE. ImageNet classification with deep convolutional neural networks. In: Pereira F, Burges CJC, Bottou L, Weinberger KQ, editors. Advances in neural information processing systems 25. Curran Associates, Inc; 2012. pp. 1097–105. http://papers.nips.cc/paper/4824-imagenet-classification-with-deep-convolutional-neural-networks.pdf.
[2] Russakovsky O, Deng J, Su H, et al. ImageNet large scale visual recognition challenge. Int J Comput Vis. 2015;115:211-52.
[3] Esteva A, Kuprel B, Novoa RA, et al. Dermatologist-level classification of skin cancer with deep neural networks. Nature. 2017;542:115-8.
[4] Data Science Bowl 2017. https://www.kaggle.com/c/data-science-bowl-2017.
[5] Wang S, Zhou M, Gevaert O, et al. A multi-view deep convolutional neural networks for lung nodule segmentation. Conf Proc IEEE Eng Med Biol Soc. 2017;2017:1752-5.
[6] Sage Bionetworks. Digital Mammography DREAM Challenge. http://sagebionetworks.org/research-projects/digital-mammography-dream-challenge/.
[7] Ribli D, Horváth A, Unger Z, et al. Detecting and classifying lesions in mammograms with deep learning. Sci Rep. 2018;8:4165.
[8] Trister AD, Buist DSM, Lee CI. Will machine learning tip the balance in breast cancer screening? JAMA Oncol. 2017;3:1463
[9] Coudray N, Ocampo PS, Sakellaropoulos T, et al. Classification and mutation prediction from non-small cell lung cancer histopathology images using deep learning. Nat Med. 2018;24:1559-67.
[10] Miotto R, Li L, Kidd BA, Dudley JT. Deep patient: an unsupervised representation to predict the future of patients from the electronic health records. Sci Rep. 2016;6:26094.
[11] Menden MP, Iorio F, Garnett M, et al. Machine learning prediction of cancer cell sensitivity to drugs based on genomic and chemical properties. PLOS One. 2013;8:e61318.